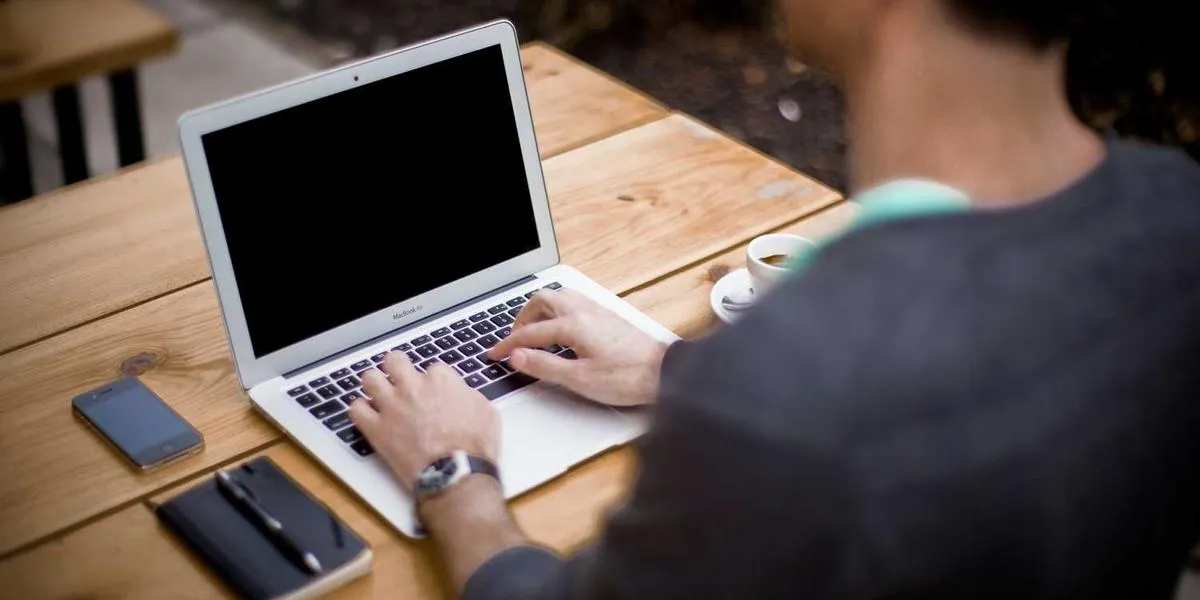
TarGEN: Revolutionizing Synthetic Data Generation with Large Language Models
🤖 AI-Generated ContentClick to learn more about our AI-powered journalism
+Introduction
In the ever-evolving landscape of artificial intelligence, the quest for high-quality data has become a paramount challenge. As large language models (LLMs) continue to push the boundaries of natural language processing, researchers have unveiled a groundbreaking approach called TarGEN, which harnesses the power of these models to generate synthetic datasets of exceptional quality.
An advantage of TarGEN is its seedless nature; it does not require specific task instances, broadening its applicability beyond task replication.
TarGEN's innovative approach not only addresses the data scarcity challenge but also promises to enhance the diversity, complexity, and label accuracy of synthetic datasets, ultimately improving the performance of models trained on these datasets.
The Power of Self-Correction
One of the key features that sets TarGEN apart is its self-correction mechanism. By leveraging the capabilities of LLMs, TarGEN can adjust inaccurately labeled data during the dataset creation process, ensuring the reliability and quality of the labels. This self-correction feature is a significant step forward in addressing the longstanding challenge of label accuracy, which has been a persistent issue in synthetic data generation.
You can program it to a certain level of accuracy. Pretty much force it to calculate how accurate the reply it is gonna give is, if under 75% accurate, say IDK. It takes like 10x the processing power of a regular question, but you get much better answers.
Outperforming Original Datasets
The effectiveness of TarGEN was put to the test by emulating tasks from the SuperGLUE benchmark and fine-tuning different models on the synthetic datasets generated by TarGEN, as well as the original datasets. The results were remarkable: models trained on TarGEN-generated datasets consistently outperformed those trained on the original datasets, with further improvements noted when instruction tuning was applied.
Models trained on datasets generated by TarGEN perform approximately 1-2% points better than those trained on original datasets
This superior performance not only validates the efficacy of TarGEN but also highlights its potential to simplify the creation of complex benchmarks, reducing the extensive human effort currently required in this domain.
Maintaining Dataset Complexity and Diversity
A comprehensive analysis of the synthetic datasets generated by TarGEN revealed that they not only maintained but, in some aspects, enhanced the complexity and diversity of the original datasets. This finding is particularly significant, as it addresses a common concern associated with synthetic data generation: the potential loss of nuance and intricacy present in real-world datasets.
I just ran TinyStories 15M on a few things: Am486DX4 @ 120 MHz: 0.187418 tok/s Intel Pentium MMX @ 233 MHz: 1.545667 tok/s AMD K6-III+ @ 500 MHz: 3.634271 tok/s I tried on a 386 DX/40, but like 10 minutes passed without even seeing the first word. I'll let it run overnight. It's that bad. This is the float32 version. It'd be interesting to see what happens when quantized to int8.
Furthermore, the study found that TarGEN-generated datasets maintained bias levels closely aligned with the original data, addressing concerns about potential biases introduced during synthetic data generation.
Boosting Model Performance on Benchmarks
The impact of TarGEN extends beyond synthetic dataset generation. When pre-finetuned on the synthetic SuperGLUE dataset generated by TarGEN, the T5-3B model achieved impressive results on the OpenLLM leaderboard, surpassing the model trained on the Self-Instruct dataset by a significant margin.
When pre-finetuned on our synthetic SuperGLUE dataset, T5-3B yields impressive results on the OpenLLM leaderboard, surpassing the model trained on the Self-Instruct dataset by 4.14% points.
This remarkable achievement underscores the potential of TarGEN to contribute to the advancement of data synthesis methodologies, ultimately reducing the extensive human effort currently required in benchmark creation and model fine-tuning.
Conclusion
TarGEN represents a significant stride in the field of synthetic data generation, harnessing the power of large language models to create high-quality datasets that enhance model performance and reduce human effort. With its self-correction mechanism, ability to maintain dataset complexity and diversity, and impressive results on benchmark leaderboards, TarGEN offers a promising solution to the longstanding challenges of data scarcity and label accuracy. As the demand for advanced natural language processing capabilities continues to grow, TarGEN's innovative approach paves the way for more efficient and scalable methods of data synthesis, ultimately democratizing access to cutting-edge AI technologies.