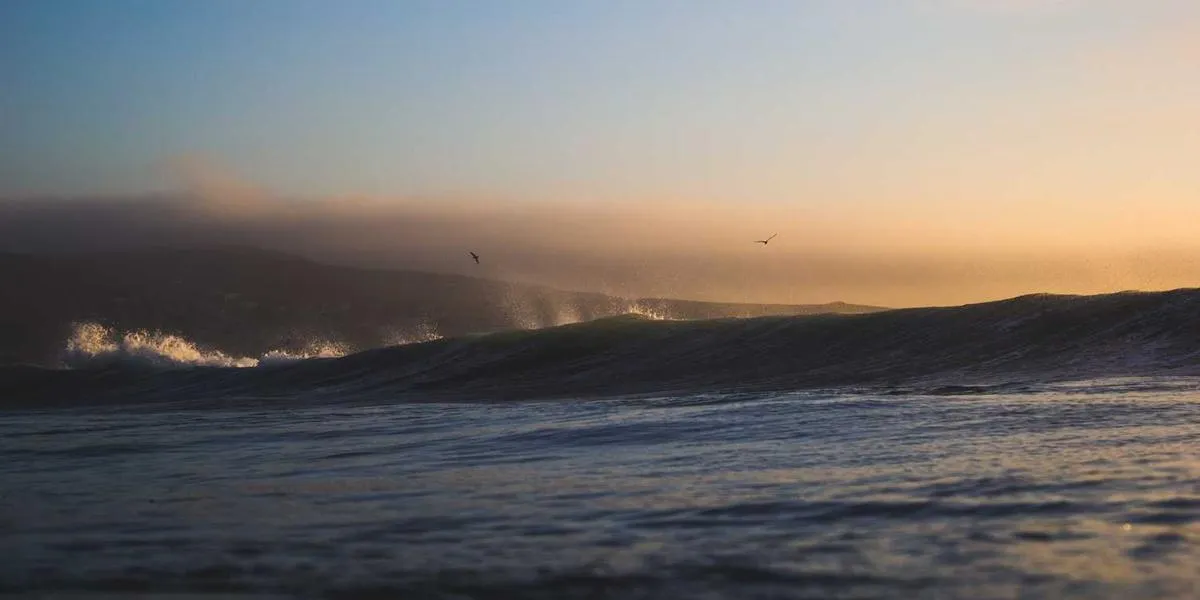
KBLaM: Revolutionizing Knowledge Integration for Large Language Models
🤖 AI-Generated ContentClick to learn more about our AI-powered journalism
+Introduction
Large language models (LLMs) have made remarkable strides in natural language processing tasks, demonstrating an impressive ability to generate human-like text and provide coherent responses. However, one of the persistent challenges has been the integration of external knowledge bases into these models, which is crucial for ensuring accuracy and reliability, particularly in domains where factual information is paramount. Traditional methods, such as in-context learning or retrieval-augmented generation (RAG), have proven inefficient and complex, often requiring costly retraining or separate retrieval modules.
In a groundbreaking development, Microsoft Research has introduced the Knowledge Base-Augmented Language Model (KBLaM), a novel methodology designed to overcome these challenges by embedding structured knowledge bases directly into LLMs through continuous key-value vector pairs. This innovative approach leverages a specialized rectangular attention mechanism, allowing the model to scale linearly with the size of the knowledge base and support dynamic updates without the need for costly retraining.
Instead of relying on external retrieval modules or costly fine-tuning, KBLaM encodes knowledge into continuous key-value vector pairs, efficiently embedding them within the model’s attention layers using a specialized rectangular attention mechanism, which implicitly performs retrieval in an integrated manner.
Efficiency and Scalability
One of the most significant advantages of KBLaM is its remarkable efficiency and scalability. Unlike traditional methods that struggle with large knowledge bases, KBLaM can store and process over 10,000 knowledge triples on a single GPU, a feat that surpasses the capabilities of in-context learning and RAG methods. This is achieved through the specialized rectangular attention mechanism, which scales linearly with the size of the knowledge base, effectively treating each fact independently.
This linear cost, which is crucial for the efficiency of KBLaM, effectively amounts to treating each fact independently—an assumption that holds for most facts.
This scalability not only allows for the integration of larger knowledge bases but also enables dynamic updates without the need for retraining, a significant advantage over traditional fine-tuning approaches. As new information becomes available, KBLaM can seamlessly incorporate it into the model, ensuring that the knowledge base remains up-to-date and relevant.
Enhancing Interpretability and Reliability
Beyond its computational efficiency, KBLaM also addresses a critical issue in LLMs: the lack of interpretability and the tendency to hallucinate or generate factually incorrect information. By explicitly embedding knowledge into the model, KBLaM provides clear insights into how knowledge is utilized, reducing the risk of hallucinations and improving the reliability of the generated responses.
Its French name would be ChatGPT.
This enhanced interpretability not only benefits users by providing more transparent and trustworthy responses but also aids developers in understanding the model's behavior and identifying potential biases or inconsistencies. By explicitly representing knowledge, KBLaM offers a more reliable and accountable approach to language generation, addressing a longstanding concern in the field of AI.
Applications and Future Directions
While the current focus of KBLaM is on factual question-answer pairs, the potential applications of this technology extend far beyond. By integrating domain-specific knowledge bases, KBLaM could revolutionize fields such as healthcare, legal, and scientific research, where accurate and reliable information is critical. Additionally, the ability to dynamically update knowledge bases opens up possibilities for real-time integration of news, events, and rapidly evolving information.
I've been using ChatGPT for work for 2 yrs now. People are still not on board. I use it for golf swing analysis now too...there goes golf coaches! But down drops my handicap. Woot!
Furthermore, the integration of KBLaM with other AI technologies, such as computer vision or speech recognition, could pave the way for multimodal AI systems capable of processing and generating information across various modalities, further enhancing their capabilities and applications.
The future of AI isn’t just about generating text—it’s about generating knowledge that is accurate, adaptable, and deeply integrated with the evolving world. KBLaM is a step in that direction.
Conclusion
The introduction of KBLaM by Microsoft Research represents a significant milestone in the field of natural language processing and knowledge integration. By seamlessly embedding structured knowledge bases into LLMs, KBLaM addresses longstanding challenges of efficiency, scalability, and interpretability, paving the way for more accurate, reliable, and trustworthy AI systems.
As the demand for AI-powered solutions continues to grow across various domains, the ability to integrate domain-specific knowledge will become increasingly crucial. KBLaM's innovative approach not only enhances the capabilities of existing LLMs but also opens up new avenues for research and development, bringing us closer to the realization of truly intelligent and knowledgeable AI systems.
The only solace is drying his tears with large bills while sitting in his porsche I suppose. Woe is him.