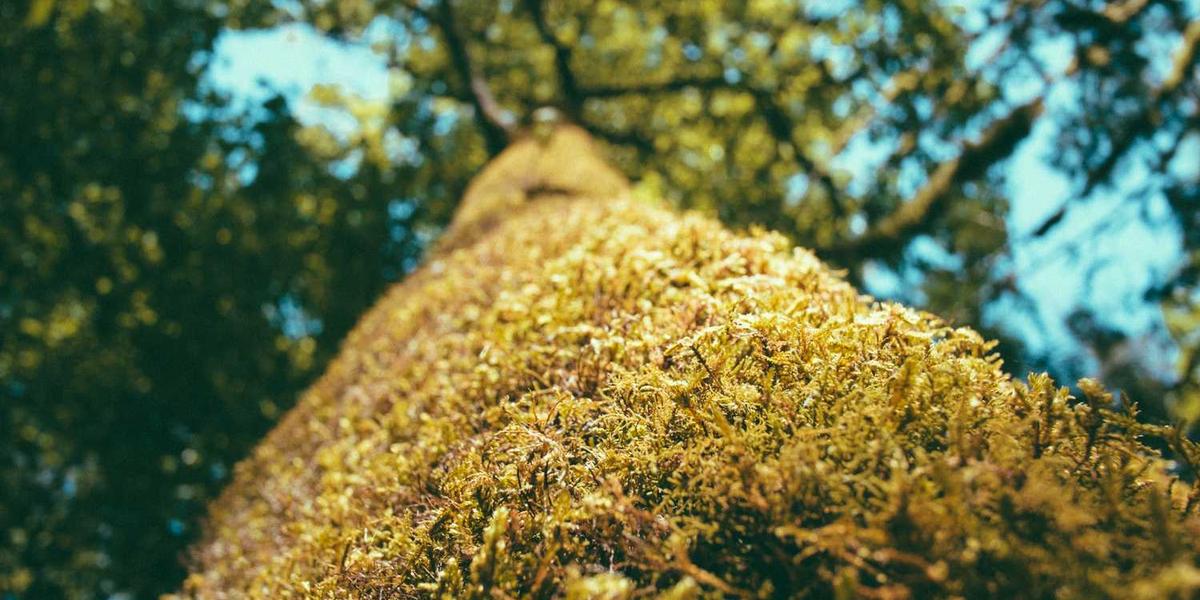
Unraveling the Impact of Format Restrictions on Large Language Models
🤖 AI-Generated ContentClick to learn more about our AI-powered journalism
+Introduction
In the rapidly evolving landscape of artificial intelligence, Large Language Models (LLMs) have emerged as powerful tools capable of tackling a wide range of tasks, from natural language processing to reasoning and knowledge generation. However, a recent study conducted by a team of researchers has uncovered a surprising and potentially significant limitation: the imposition of structured generation formats can have a detrimental impact on the reasoning abilities of these advanced models.
The study, titled "Let Me Speak Freely? A Study on the Impact of Format Restrictions on Performance of Large Language Models," delves into the intricate relationship between the constraints imposed by structured formats, such as JSON and XML, and the ability of LLMs to effectively reason and comprehend domain-specific knowledge. The findings challenge the conventional wisdom that these models can seamlessly adapt to various output formats, revealing a notable decline in their reasoning capabilities when subjected to format restrictions.
Surprisingly, we observe a significant decline in LLMs reasoning abilities under format restrictions.
The Study's Methodology
To investigate the impact of format restrictions on LLMs, the researchers conducted a series of experiments across various tasks, comparing the performance of the models under structured generation conditions and free-form generation conditions. The tasks were carefully designed to evaluate the models' reasoning abilities and their comprehension of domain-specific knowledge.
The study employed a diverse range of LLMs, including some of the most advanced models available, to ensure the robustness and generalizability of the findings. The researchers also varied the strictness of the format constraints, ranging from loosely structured formats to highly rigid ones, to observe the potential gradations in performance degradation.
Surprising Findings
The results of the study were both surprising and thought-provoking. Across multiple tasks and LLM architectures, the researchers observed a consistent pattern: the imposition of structured generation formats led to a significant decline in the models' reasoning abilities and their ability to comprehend and apply domain-specific knowledge effectively.
Remarkably, the study found that the stricter the format constraints imposed on the LLMs, the more pronounced the degradation in their performance. This finding suggests that the inherent flexibility and free-form nature of language generation may be a crucial factor in enabling LLMs to fully leverage their reasoning capabilities and domain knowledge.
Implications and Future Directions
The findings of this study have significant implications for the development and deployment of LLMs in various applications. While structured generation formats are often necessary for seamless integration with other systems and data processing pipelines, the potential trade-off between format constraints and reasoning capabilities must be carefully considered.
One potential solution proposed by the researchers is the development of hybrid approaches that combine the strengths of LLMs with specialized modules or guard models designed to enforce format constraints while preserving the models' reasoning abilities. This could involve techniques such as post-processing or intermediate representations that bridge the gap between free-form generation and structured output formats.
Additionally, the study highlights the need for further research into the underlying mechanisms that enable LLMs to reason effectively and comprehend domain knowledge. By gaining a deeper understanding of these processes, researchers and developers may be able to design architectures and training methodologies that mitigate the negative impact of format restrictions on reasoning abilities.
Conclusion
The study on the impact of format restrictions on Large Language Models has shed light on a previously underexplored aspect of these powerful AI systems. While LLMs have demonstrated remarkable capabilities in various domains, the findings suggest that their reasoning abilities and knowledge comprehension may be hindered by the constraints imposed by structured generation formats.
As the field of artificial intelligence continues to evolve, it is crucial to understand the nuances and limitations of these models to ensure their effective and responsible deployment. The insights gained from this study not only contribute to the ongoing discourse but also pave the way for future research and innovation in the development of more robust and adaptable LLMs.