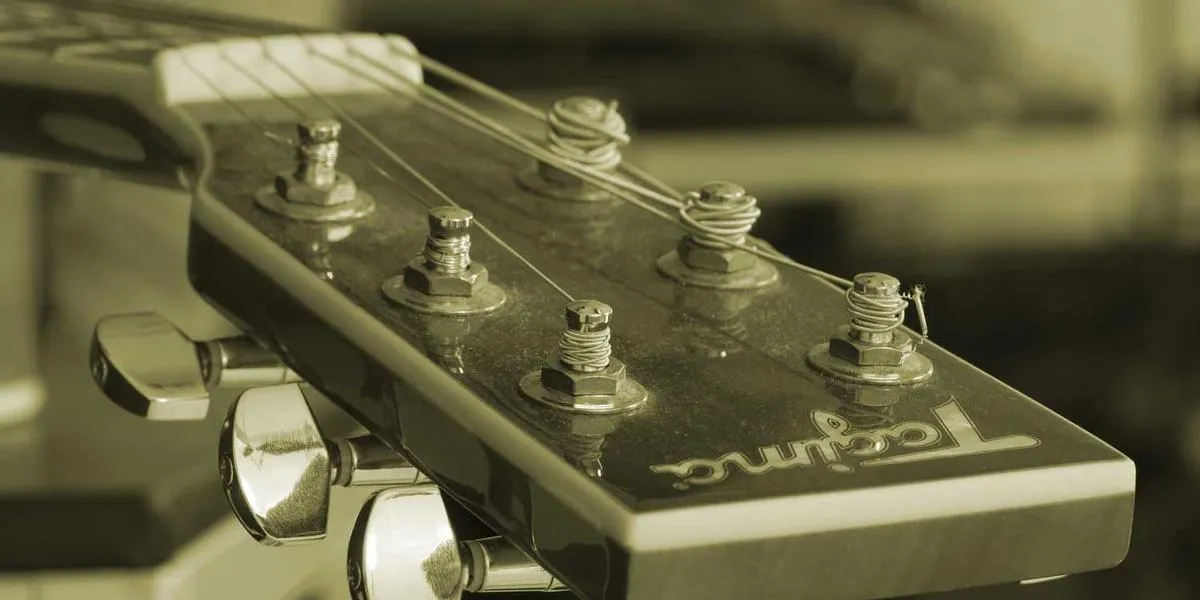
Gemma 3 QAT: Pushing the Boundaries of Efficient AI Inference
🤖 AI-Generated ContentClick to learn more about our AI-powered journalism
+Introduction
In the rapidly evolving world of artificial intelligence, the pursuit of efficient and accessible AI models has become a paramount objective. As the demand for AI applications continues to soar across various industries, the need for models that can deliver high-quality performance while minimizing computational requirements has never been more pressing. Enter Google's Quantized Aware Training (QAT) models, a groundbreaking approach that promises to reshape the landscape of AI inference.
The Quantization Conundrum
Traditionally, AI models have been trained and deployed using high-precision floating-point representations, which can result in significant computational demands and memory requirements. This limitation has posed a significant challenge for deploying AI models on resource-constrained devices or in environments where computational power is scarce. Quantization, the process of reducing the precision of model parameters, has emerged as a potential solution to this problem. However, naive quantization techniques often lead to a substantial degradation in model performance, rendering the resulting models impractical for real-world applications.
Quantized Aware Training: A Game-Changer
Google's Quantized Aware Training (QAT) approach represents a significant breakthrough in addressing the quantization conundrum. Unlike traditional quantization methods, QAT incorporates quantization into the training process itself, allowing the model to adapt and optimize its parameters for low-precision representations. This innovative technique results in quantized models that can achieve performance levels comparable to their full-precision counterparts, while significantly reducing the computational and memory requirements.
QAT is expected to be better, but figured while I'm at it I might as well make others to see what happens?
One of the most promising QAT models to emerge is Google's Gemma 3, a 27-billion parameter language model that has been quantized using the QAT approach. Early experiments and benchmarks have demonstrated Gemma 3's remarkable ability to deliver high-quality performance even at lower quantization levels, such as Q2 (2-bit quantization), which significantly reduces the model's memory footprint and computational requirements.
I wanted to test how well QAT models do at a lower quant size so I grabbed the smallest quant currently out for it, Q2\_K at 10.5 GB. [https://huggingface.co/bartowski/google\_gemma-3-27b-it-qat-GGUF] I use my models mostly for my Japanese indie game, so following instructions, custom formatting and if it can roleplay or not is what I look for in models. My tests were all done in Japanese, which many models already have issues with at Q4 so I mostly use Q5. In my testing there were no grammatical errors, no random English or Chinese characters. It was able to roleplay in a custom format where I split the spoken words, the actions and the thoughts of the character into different brackets like ()<>「」without any issues. I also asked it basic questions about celebrities, and historical events, it got names and basic information right but dates were all wrong. My tests were done in Ollama with the standard Gemma3 settings. Overall I am really impressed by the performance of the model especially for being a 27B at Q2. In theory running a 70B model at Q2 would fit into a single 24GB GPU so this technology is very interesting and could allow us to fit even larger models into our cards. After testing it I am really excited for more QAT models to come out in the future.
Expanding the Horizons of AI Accessibility
The implications of QAT models like Gemma 3 extend far beyond mere computational efficiency. By reducing the resource requirements for deploying AI models, QAT opens up new avenues for democratizing AI technology and making it accessible to a broader range of users and applications. Researchers, developers, and enthusiasts can now explore and experiment with state-of-the-art language models on consumer-grade hardware, fostering innovation and accelerating the pace of AI development.
We are living in the future
Moreover, the reduced computational requirements of QAT models could pave the way for deploying AI on edge devices and resource-constrained environments, enabling a wide range of applications in areas such as Internet of Things (IoT), mobile computing, and embedded systems. This democratization of AI technology has the potential to catalyze innovation across various industries, from healthcare and finance to agriculture and manufacturing.
Collaborative Efforts and Open-Source Initiatives
The development and dissemination of QAT models like Gemma 3 have been driven by a collaborative effort involving Google, the open-source community, and various research institutions. Google's decision to release the QAT weights for Gemma 3 has enabled researchers and developers to explore and build upon this groundbreaking technology.
No, we just released half precision QATs corresponding to Q4\_0 and folks went ahead with quantizing to Q4\_0. Prince, our MLX collaborator, found that the 3 bit quants were also working better than naive 3 bit quants, so he went ahead to share those as well We'll follow up with LM Studio, thanks!
Open-source initiatives and collaborations have played a crucial role in the rapid development and adoption of QAT models. Projects like MLX, llama.cpp, Ollama, and LM Studio have contributed significantly to the quantization and deployment of Gemma 3, enabling researchers and developers to experiment with and optimize the model for various use cases.
Addressing Privacy and Security Concerns
While the potential benefits of QAT models are undeniable, their widespread adoption also raises concerns regarding privacy and security. As AI models become more accessible and deployable on a broader range of devices, the risk of data breaches and unauthorized access to sensitive information increases. Addressing these concerns will be crucial for ensuring the responsible and ethical use of QAT technology.
Nope, this is a true invasion of privacy and not necessary. I'll use alternatives, some of which are performing well beyond OpenAI's models and have better value for money.
Wtf 🤣. Dystopian shit. Sama really wants all the biometric info, first Worldcoin and now this. Fuck sama and closed ai.
As the adoption of QAT models accelerates, it will be crucial for developers, researchers, and organizations to prioritize robust security measures, such as encryption, access controls, and secure deployment practices. Additionally, the development of privacy-preserving AI techniques and the establishment of clear ethical guidelines will be essential to ensure the responsible and trustworthy use of these powerful technologies.
Conclusion
Google's Quantized Aware Training (QAT) approach, exemplified by the Gemma 3 language model, represents a significant milestone in the pursuit of efficient and accessible AI. By delivering impressive performance at lower computational costs, QAT models are poised to democratize AI technology and unlock new avenues for innovation across various industries. However, as with any transformative technology, the widespread adoption of QAT models will require a concerted effort to address privacy and security concerns, ensuring that the benefits of this groundbreaking approach are realized in a responsible and ethical manner.