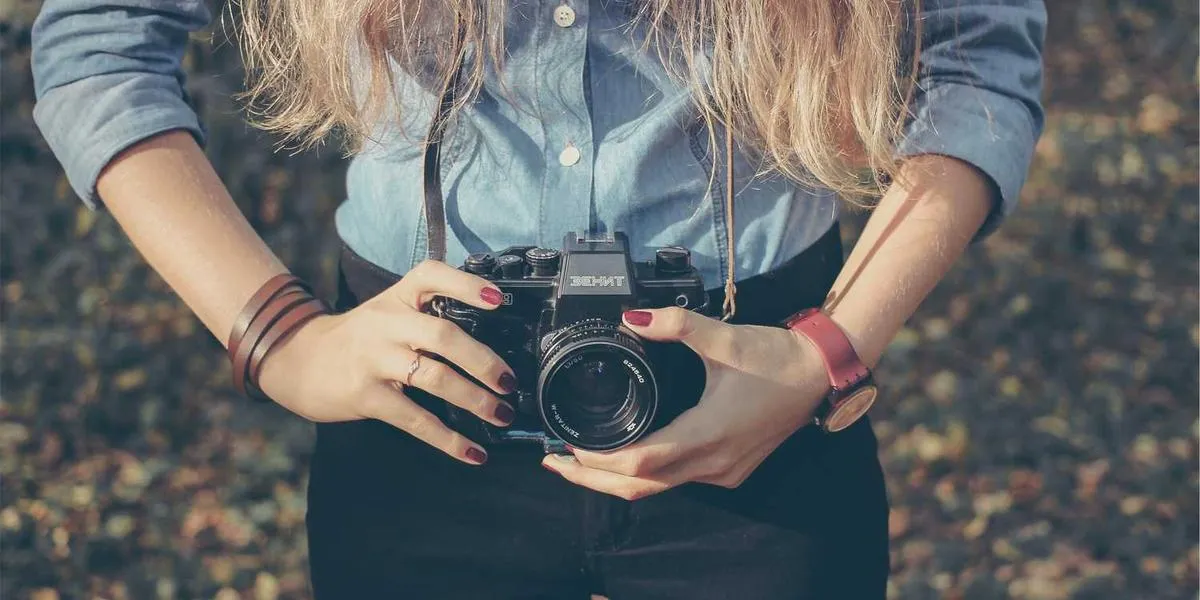
Eureka ML Insights: Microsoft's Framework for Comprehensive Model Evaluation
🤖 AI-Generated ContentClick to learn more about our AI-powered journalism
+Introduction
In the rapidly evolving landscape of artificial intelligence, the evaluation of large language models has become a critical challenge. As these models grow in size and complexity, traditional single-metric assessments often fail to capture the nuances of their capabilities. Recognizing this limitation, Microsoft has introduced the Eureka ML Insights framework, an open-source project designed to facilitate comprehensive and reproducible evaluations of generative models.
A framework for standardizing evaluations of large foundation models, beyond single-score reporting and rankings.
Eureka ML Insights emphasizes efficiency and flexibility, allowing researchers and practitioners to configure customizable evaluation pipelines tailored to their specific needs. By providing a structured approach to model evaluation, the framework aims to foster a more comprehensive understanding of model capabilities across a wide range of tasks and benchmarks.
Comprehensive Evaluation Pipelines
One of the key strengths of Eureka ML Insights lies in its support for a diverse array of benchmarks and metrics. The framework includes pre-defined evaluation pipelines for tasks ranging from geometric reasoning and toxicity detection in text to image-to-text and text-to-text evaluations. These pipelines incorporate benchmarks such as GeoMeter, MMMU, and FlenQA, among others, enabling researchers to evaluate models across multiple dimensions.
Small MoE and 8B are coming? Nice! Finally some good sizes you can run on lower end machines that are still being capable.
The framework's flexibility extends beyond its support for diverse benchmarks. Researchers can configure various components of the evaluation pipeline, including data processing, inference, and evaluation reporting. This level of customization allows for tailored experiments and facilitates the exploration of specific research questions or use cases.
Fostering Reproducibility and Community Contributions
Reproducibility is a cornerstone of scientific research, and Eureka ML Insights embraces this principle. The framework provides detailed guidelines and support for reproducing experiments, ensuring that researchers can validate and build upon each other's work. This commitment to reproducibility not only enhances the credibility of the research but also accelerates progress by enabling efficient knowledge sharing and collaboration.
Moreover, Eureka ML Insights encourages community contributions, recognizing the collective power of diverse perspectives and expertise. Researchers can contribute new benchmarks or modify existing ones, fostering a collaborative ecosystem that drives innovation and expands the framework's capabilities. This open-source approach aligns with Microsoft's commitment to advancing the field of artificial intelligence through shared knowledge and resources.
Implications and Future Directions
The introduction of Eureka ML Insights represents a significant step towards more comprehensive and nuanced evaluations of large language models. By moving beyond simplistic single-metric assessments, the framework promises to provide a deeper understanding of model capabilities, limitations, and potential areas for improvement.
this aint a scene, its a god damn arms race 🎵
As the field of artificial intelligence continues to evolve at a rapid pace, frameworks like Eureka ML Insights will play a crucial role in guiding the development and deployment of these powerful models. By fostering a more nuanced understanding of model capabilities, researchers and practitioners can make informed decisions, mitigate potential risks, and ensure that these technologies are aligned with ethical principles and societal values.
Looking ahead, the Eureka ML Insights framework holds promise for further advancements and applications. As models become increasingly complex and multi-modal, the need for comprehensive evaluation frameworks will only grow. Additionally, the framework's emphasis on community contributions and extensibility positions it as a valuable resource for driving collaborative research and innovation in the field of artificial intelligence.
Conclusion
Microsoft's Eureka ML Insights framework represents a significant step forward in the evaluation of large language models. By offering a structured approach to comprehensive model assessment, the framework addresses a critical need in the field of artificial intelligence. As researchers and practitioners continue to push the boundaries of what is possible with these powerful models, frameworks like Eureka ML Insights will play a vital role in ensuring that their development and deployment are guided by a deep understanding of their capabilities and limitations.