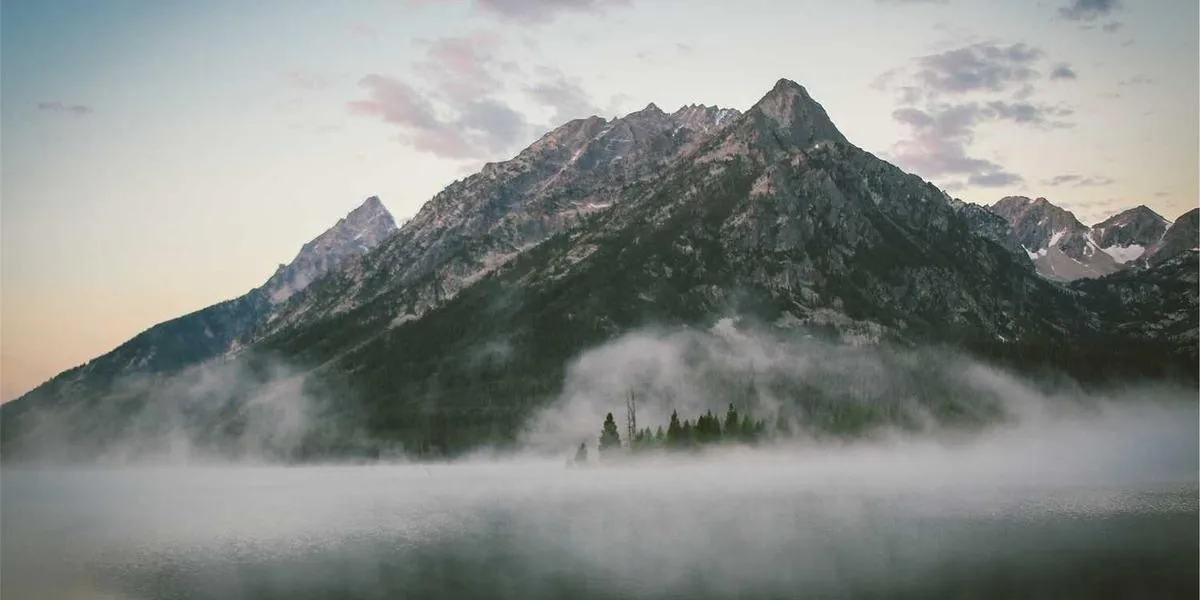
The Candle Test: Exposing Limitations in Language Models' Reasoning Abilities
🤖 AI-Generated ContentClick to learn more about our AI-powered journalism
+The Candle Test: A Deceptively Simple Challenge
In the ever-evolving landscape of artificial intelligence, language models have emerged as a powerful tool, capable of understanding and generating human-like text with remarkable fluency. However, a seemingly innocuous thought experiment known as the 'Candle Test' has exposed a fundamental limitation in these models' ability to reason abstractly and generalize beyond their training data.
The Candle Test, first proposed by psychologist Karl Duncker in 1945, presents a simple scenario: You are given a box of thumbtacks, a candle, and a book of matches. Your task is to attach the candle to the wall in such a way that it can be lit without dripping wax onto the table or floor. The solution requires using the box as a platform to hold the candle, with the thumbtacks securing it to the wall – a creative approach that involves repurposing the objects in an unconventional manner.
Language Models' Struggle with Abstract Reasoning
While the Candle Test may seem trivial to humans, it has proven to be a formidable challenge for language models, even those considered to be at the cutting edge of AI technology. In a recent discussion on the /r/LocalLLaMA subreddit, users shared their experiences with various language models, and the results were eye-opening.
I'm not aware of any Open Weights models passing the test~~ (I'm stupid - Mistrals) from closed ones - Sonnet 3.5, Opus 3, GPT 4.5 are the ones that do. I do have plenty more tasks like this one, so I'll let this one slip into training :)
The comment highlights the struggle faced by many language models, both open-source and proprietary, in solving the Candle Test. Even models considered to be among the most advanced, such as GPT-4.5 and Sonnet 3.5, initially failed to provide the correct solution, demonstrating a lack of generalization and abstract reasoning capabilities.
The Implications for AI Development
The Candle Test's ability to expose the limitations of language models has significant implications for the future development of AI systems. As these models continue to advance and find applications in various domains, their inability to reason abstractly and generalize beyond their training data could pose significant challenges.
Many real-world distribution shifts are not represented in standard domain adaptation datasets and prior empirical work has shown that domain adaptation methods developed using these standard datasets may not generalize well to real-world distribution shifts.
As highlighted in the quote from the OpenReview article, the inability of language models to generalize beyond their training data can lead to significant performance degradation when deployed in real-world scenarios. This limitation could hinder the adoption of AI systems in critical domains, such as healthcare, finance, and decision-making processes, where abstract reasoning and the ability to handle novel situations are essential.
Addressing the Generalization Challenge
To address the generalization challenge exposed by the Candle Test, researchers and developers are exploring various approaches. One promising direction is the development of new architectures and training methodologies that can better capture abstract reasoning and generalization capabilities.
Oh yeah, this is huge news. We desperately need a different architecture than transformers. Transformers is still king, but I really wanna see how far you can take this architecture.
As evidenced by the Reddit comment, there is a growing recognition within the AI community that alternative architectures beyond the widely used transformer models may be necessary to achieve true generalization and abstract reasoning capabilities. Approaches like the diffusion-based Dream 7B model, developed by the University of Hong Kong, are being explored as potential solutions to this challenge.
Conclusion
The Candle Test has proven to be a valuable tool in exposing the limitations of current language models, particularly in the realm of abstract reasoning and generalization. While these models have achieved remarkable feats in natural language processing and understanding, their inability to solve seemingly simple problems like the Candle Test highlights the need for continued research and development. As AI systems become increasingly integrated into various aspects of our lives, addressing these limitations will be crucial to ensuring their reliability, safety, and effectiveness in real-world scenarios. The path forward may involve exploring alternative architectures, developing new training methodologies, or a combination of both. Ultimately, the Candle Test serves as a humbling reminder that, despite the remarkable progress in AI, there is still much work to be done to achieve true artificial general intelligence.