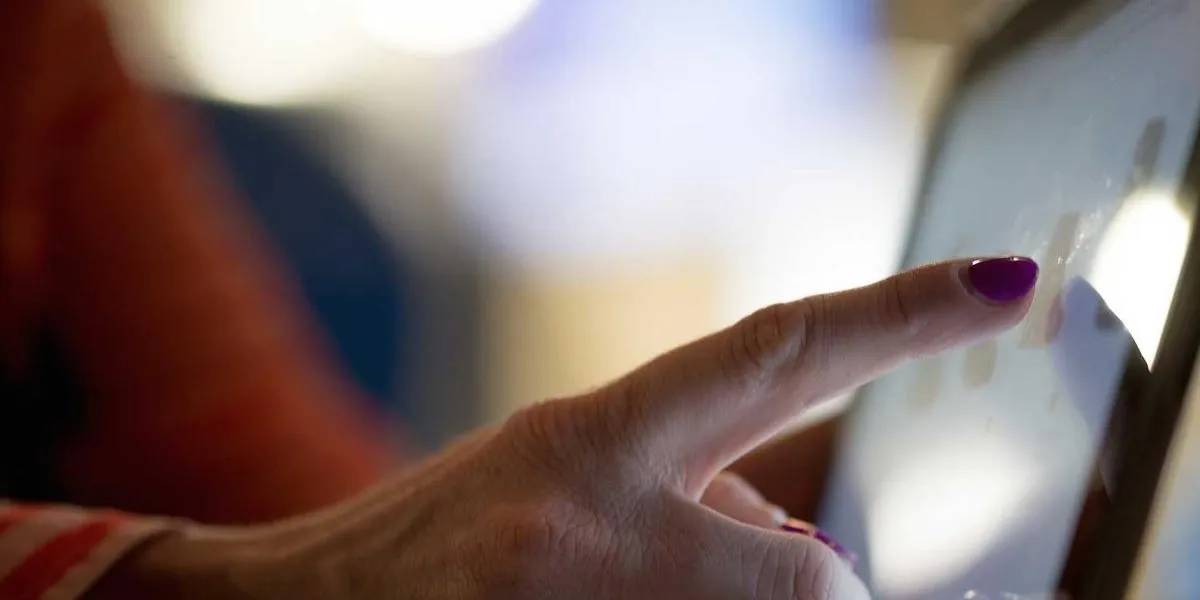
ACT-R: The Preeminent Framework for Understanding Human Cognition
๐ค AI-Generated ContentClick to learn more about our AI-powered journalism
+Introduction
In the vast landscape of cognitive theories, one framework stands out as the most comprehensive and well-validated model for understanding human cognition: the ACT-R (Adaptive Control of Thought-Rational) theory. Developed by John R. Anderson and his colleagues, ACT-R has garnered significant attention and praise for its ability to explain a wide range of cognitive phenomena, closely match experimental data, and offer a detailed account of functional neuroanatomy.
There are many theories of cognition. But if you want to work within a framework with the following properties: Explains the major cognitive phenomena we know about. Fits experimental data well, down to human reaction times, in a wide variety of psychological experiments. Has a relatively complete story about functional neuroanatomy. Well, then, I'm not aware of any theories which fit the bill as well as ACT-R theory.
The Strengths of ACT-R
One of the key strengths of ACT-R lies in its ability to replicate human behavior across a diverse range of psychological tests, including accurately predicting reaction times. This level of precision and alignment with experimental data sets ACT-R apart from many other cognitive theories. Additionally, ACT-R provides a comprehensive account of functional neuroanatomy, offering insights into the underlying neural mechanisms that drive cognitive processes.
I very much agree with the summary in the main post here. ACT-R is the best current model if you are trying to match human experimental data, including things like accuracy and reaction times. And it's been applied to a much wider variety of tasks than any other competing theory.
Limitations and Considerations
While ACT-R's ability to replicate human performance in psychological tests is impressive, it is important to note that it is not an artificial general intelligence (AGI) system. As abramdemski clarifies, "Although it is supposed to be a computational model of cognition, ACT-R is not an AGI. I would say it's not even a proto-AGI." This distinction highlights ACT-R's focus on modeling human cognitive processes rather than creating a general artificial intelligence.
Additionally, while ACT-R's ability to fit experimental data is impressive, some critics argue that its approach of introducing new mechanisms to account for new data could be seen as overfitting. It is important to acknowledge that not all components of ACT-R may be entirely accurate representations of the underlying cognitive processes.
Potential Applications
Despite its limitations, ACT-R's strengths in modeling human cognition and behavior make it a valuable tool for various applications. One area where ACT-R could prove beneficial is in understanding human irrationality and biases. By replicating these cognitive phenomena, researchers could gain insights into the underlying mechanisms and potentially develop strategies to mitigate irrational tendencies.
Additionally, ACT-R's ability to model human decision-making processes could be leveraged in the field of inverse reinforcement learning. By understanding the cognitive processes that drive human behavior, researchers could potentially develop AI systems that can infer the underlying reward functions and decision-making strategies employed by humans.
Furthermore, ACT-R's insights into functional neuroanatomy could inform the development of neuroanatomy-inspired AI architectures, potentially leading to more human-like cognitive capabilities in artificial systems.
Conclusion
While the field of cognitive science is vast and diverse, the ACT-R theory stands out as a comprehensive and well-validated framework for understanding human cognition. Its ability to replicate experimental data, account for functional neuroanatomy, and provide insights into cognitive processes make it a valuable tool for researchers and practitioners alike. Whether exploring human irrationality, developing AI alignment techniques, or informing neuroanatomy-inspired AI architectures, ACT-R offers a robust foundation for advancing our understanding of the human mind and its implications for artificial intelligence.
See also
- ๐ [Cognitive architectures](https://www.google.com/search?q=cognitive+architectures+for+modeling+human+cognition) provide frameworks for understanding and simulating human cognitive processes.
- ๐ง [Functional neuroanatomy](https://www.google.com/search?q=functional+neuroanatomy+and+cognitive+processes) explores the relationship between brain structures and cognitive functions.
- ๐ [Inverse reinforcement learning](https://www.google.com/search?q=inverse+reinforcement+learning+for+modeling+human+behavior) aims to infer the reward functions and decision-making strategies employed by humans.
You may also enjoy
- ๐งช [Cognitive psychology experiments](https://www.google.com/search?q=cognitive+psychology+experiments+and+tasks) provide insights into various cognitive processes and serve as benchmarks for cognitive models.
- ๐ค [Neuromorphic computing](https://www.google.com/search?q=neuromorphic+computing+and+brain-inspired+architectures) explores the development of computing systems inspired by the structure and function of the human brain.